Understanding the Importance of Image Datasets for Object Detection
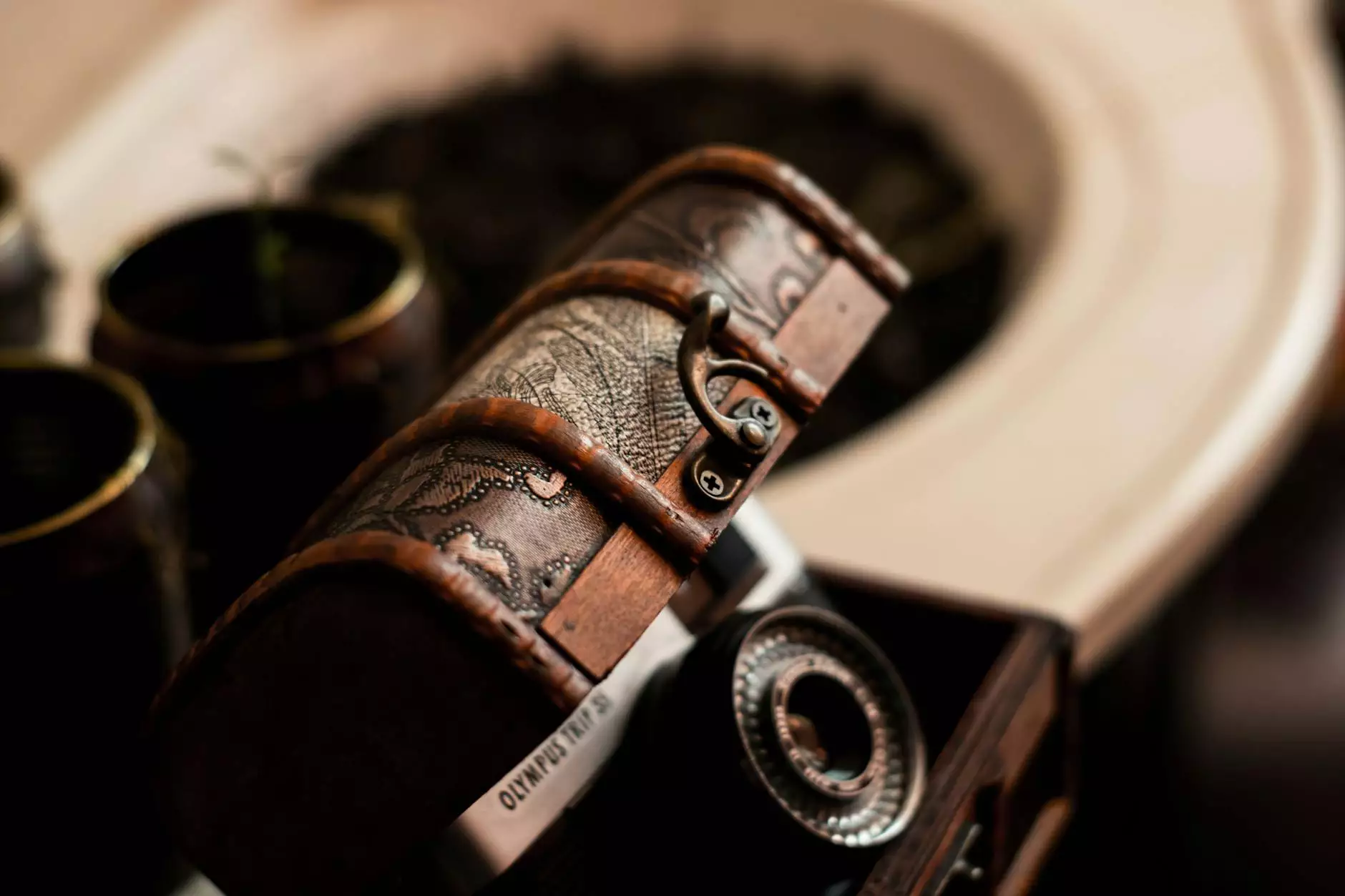
The advancement of artificial intelligence, particularly in the realms of computer vision and object detection, has revolutionized numerous industries. Creating successful models relies heavily on image datasets for object detection, which serve as the foundation for training and refining algorithms. This article will delve deep into the nuances of image datasets, the role they play in data annotation platforms, and how they help businesses achieve remarkable innovations.
The Core of Object Detection: Image Datasets
At its essence, object detection is the task of identifying and localizing objects within an image. The accuracy of these models is largely determined by the quality and comprehensiveness of the image dataset used for training. Here, we discuss some vital aspects of image datasets:
What Constitutes an Effective Image Dataset?
An effective image dataset for object detection should include a variety of images that encapsulate the different scenarios where the target objects may appear. Some key characteristics include:
- Diversity: The dataset should encompass a wide range of backgrounds, lighting conditions, and object orientations.
- Annotation Quality: Each image should be properly annotated with bounding boxes or segmentation masks that accurately define the objects' presence.
- Size: A larger dataset can provide more training opportunities, helping the model generalize better to unseen data.
- Relevance: Images must represent the particular objects relevant to the end application.
Challenges in Creating Image Datasets
Despite the importance of high-quality image datasets for successful object detection, several challenges can arise during the dataset creation process:
- Data Collection: Sourcing images that meet the requirements can be time-consuming and resource-intensive.
- Labeling Efforts: Manual annotation can introduce errors, making it essential to adopt tools that ensure accuracy.
- Scalability: As the volume of data increases, managing and maintaining datasets can become increasingly complicated.
Advantages of Using a Data Annotation Platform
To address the challenges of creating high-quality image datasets for object detection, leveraging a data annotation platform like KeyLabs.ai can be immensely beneficial. Here are some advantages:
- Streamlined Process: Data annotation platforms offer automated tools that save time and reduce human error.
- Collaboration Tools: Facilitates teamwork, allowing multiple annotators to work on projects simultaneously.
- Scalable Solutions: Easily manage large datasets without the loss of quality or consistency.
- Cost-Effective: Reduces the overall cost associated with data preparation, enabling businesses to focus on model development.
The Role of Data Annotation in Object Detection
Data annotation is critical for training effective object detection models. It involves tagging images with information that machine learning algorithms use to learn to identify and locate objects. Let's explore some common methods:
Types of Data Annotation Techniques
Various methods of annotation can be employed depending on the use case and complexity of the images:
- Bounding Box: This technique involves drawing rectangles around the objects of interest, ideal for detecting individual objects in images.
- Semantic Segmentation: This method labels each pixel in an image, useful for applications where precise delineation of object boundaries is necessary.
- Instance Segmentation: This is an advanced version of semantic segmentation that differentiates between separate objects of the same category.
- Keypoint Annotation: It involves identifying specific points on an object, often employed in pose estimation tasks.
Best Practices for Data Annotation
To maximize the efficiency and effectiveness of your annotation process, consider the following best practices:
- Clear Guidelines: Develop comprehensive guidelines for annotators to ensure consistency and accuracy.
- Quality Control: Implement a review system where annotated data is periodically checked for errors.
- Use of Automation: Employing AI-assisted tools can expedite the process and enhance the quality of annotations.
- Regular Training: Continually training annotators on best practices improves the quality of results.
Building a Quality Image Dataset for Object Detection
Creating a high-quality image dataset for object detection requires strategic planning and execution. Below is a streamlined approach to building your dataset:
Step 1: Define Your Objective
Before you begin, clearly define your project objectives. What objects do you need to detect? What variations must be addressed? Understanding your goals helps in effectively curating your dataset.
Step 2: Collect Data
Utilize various sources such as:
- Stock photo websites
- Public datasets available for research
- Your own image capture methods
- Crowdsourcing platforms for additional data collection
Step 3: Annotate Your Data
Use a robust data annotation tool like KeyLabs.ai to ensure meticulous and fast annotation. Choose the annotation type that aligns with your objectives.
Step 4: Organize and Clean Your Dataset
Make sure your dataset is well-structured. Keep images organized in folders according to categories, and remove any duplicates or irrelevant images. Ensure all annotations are accurate to avoid future problems during model training.
Evaluating Your Dataset
Once your dataset is created, thorough evaluation is paramount to ensure its suitability for training models. Key metrics include:
Dataset Quality Assessment
Consider the following metrics:
- Completeness: Are all necessary objects represented?
- Diversity: Does the dataset cover various conditions and angles?
- Annotation Accuracy: Are the annotations precise and reliable?
Using Validation Sets
Creating a separate validation dataset enables you to test your model's performance and further refine your image dataset.
Future Trends in Image Datasets for Object Detection
As technology advances, the field of object detection will continue to evolve. Here are some trends to look out for:
Increased Use of Synthetic Data
The growth of generative models enables the creation of synthetic images, which can supplement real datasets and help overcome data scarcity issues.
Focus on Real-Time Annotation
As demand for rapid turnaround increases, real-time annotation solutions will become essential, especially in dynamic environments such as autonomous driving.
Integration of Advanced AI Tools
With AI tools evolving, future annotation platforms will likely integrate deeper learning models to assist and automate the data preparation process further.
Conclusion
In conclusion, image datasets for object detection are an invaluable resource that can drastically influence the effectiveness of machine learning models. Businesses looking to leverage the power of object detection must prioritize the quality and diversity of their datasets while considering the integration of advanced data annotation platforms like KeyLabs.ai. By adopting best practices and remaining informed of future trends, organizations can fuel their growth and gain a competitive edge in the market.
By following the insights and guidelines outlined in this article, you can effectively build and utilize image datasets that empower your machine learning initiatives and lead to groundbreaking advancements in your business domain.