The Future of Agriculture: Leveraging Machine Learning with Quality Datasets
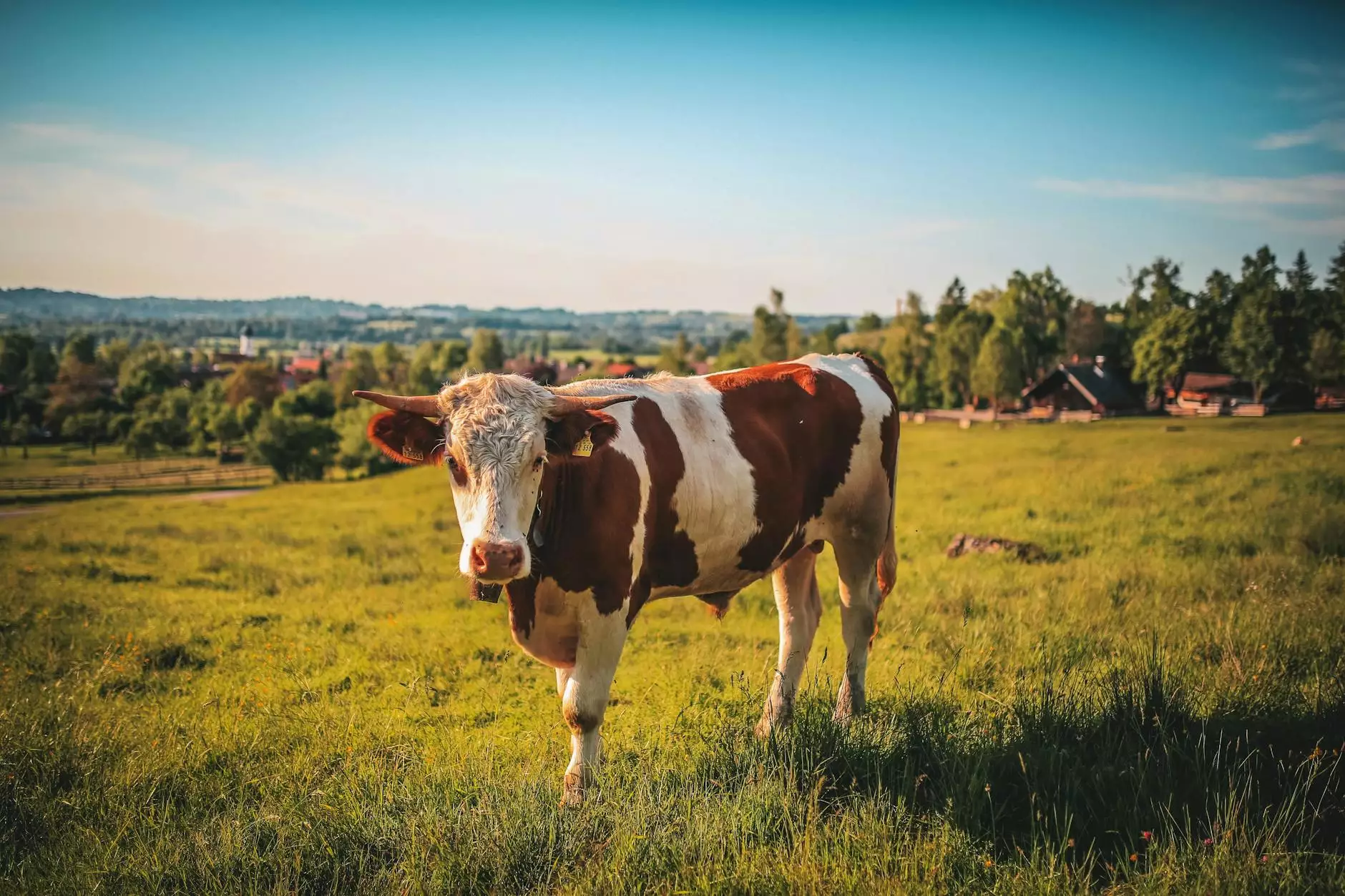
In today’s ever-evolving landscape, agriculture is reaching new heights with the integration of machine learning. The incorporation of advanced technologies in farming has led to unprecedented opportunities for efficiency and productivity. A crucial element in this technological transformation is the availability of high-quality agriculture datasets for machine learning. This article delves into the various dimensions of how these datasets can empower farmers and agribusinesses alike, enhancing decision-making processes and agricultural practices.
Understanding Agriculture Datasets for Machine Learning
Agriculture datasets constitute a collection of relevant data that can be utilized by machine learning algorithms to recognize patterns, make predictions, and drive insights that are vital for modern farming practices. These datasets can range from soil characteristics, weather patterns, crop yields, pest infestations, to market conditions. Each of these data points can be crucial in building machine learning models that help in optimizing agricultural outputs.
Types of Agriculture Datasets
There are numerous types of datasets that can be employed in agriculture machine learning models. Each type can significantly impact the predictive accuracy and operational efficiency:
- Soil Data: Information about soil types, composition, nutrient levels, and moisture content.
- Satellite Imagery: High-resolution images used for monitoring crop health, growth stages, and land use.
- Weather Data: Historical and forecasted weather conditions, including temperature, rainfall, and humidity.
- Crop Yield Data: Historical yield information across different regions and conditions.
- Pest and Disease Data: Patterns of pest populations and disease outbreaks that impact crops.
- Market Data: Information regarding crop prices, demand trends, and economic factors affecting agriculture.
Why Quality Matters in Agriculture Datasets
The quality of the datasets used in machine learning can significantly influence the performance of the resulting models. High-quality datasets must be accurate, complete, consistent, and up-to-date. Let’s explore each of these qualities:
Accuracy
Accurate datasets ensure that the machine learning models built upon them can provide reliable predictions. For instance, if the data reflects incorrect crop yields or soil quality, the insights derived may lead to poor farming decisions.
Completeness
A complete dataset encompasses all necessary factors influencing agricultural outcomes. Incomplete data can limit the model’s ability to learn and generalize, potentially resulting in suboptimal recommendations.
Consistency
Data should be consistently collected and curated. Inconsistencies, such as varying units of measurement or conflicting data points, can confuse machine learning algorithms and degrade their effectiveness.
Up-to-date
Given the dynamic nature of agriculture influenced by climate change and technological advancements, it is imperative to utilize the most current datasets available. Outdated data can lead to misguided strategies and losses.
The Role of Machine Learning in Agriculture
Machine learning can revolutionize agricultural practices by enabling data-driven decision-making. Here are some significant applications:
Precision Agriculture
With machine learning models powered by high-quality agriculture datasets, farmers can adopt precision agriculture techniques. This involves monitoring and managing field variability in soil composition and crop health, which leads to targeted interventions. For example, machine learning techniques can determine the optimal amount of fertilizers or pesticides needed, reducing waste and increasing yields.
Yield Prediction
Accurate crop yield predictions are vital for effectively planning the growing season. Using historical yield data combined with weather forecasts and soil conditions, machine learning models can provide farmers with more accurate estimates of expected yields, aiding in inventory management and market planning.
Pest and Disease Management
Through the analysis of historical pest data and environmental variables, machine learning systems can help predict pest outbreaks or disease occurrences. Early detection allows farmers to take preventive measures, reducing crop losses and improving sustainability.
Best Practices for Collecting Agriculture Datasets
Collecting high-quality datasets for machine learning in agriculture involves certain best practices:
- Standardization: Use consistent formats and units for data collection to ensure uniformity.
- Automated Data Gathering: Utilize IoT devices, sensors, and drones to automate data capture for real-time insights.
- Collaborative Efforts: Engage with other farmers, agricultural organizations, and research institutions to share data and insights.
- Regular Updates: Frequently update datasets to reflect current conditions and trends in agriculture.
Challenges in Utilizing Agriculture Datasets
While there are significant benefits to using agriculture datasets for machine learning, several challenges persist:
Data Privacy Concerns
As with any data-related field, privacy concerns can emerge when dealing with sensitive agricultural data. Farmers may be hesitant to share data if they fear it may be misused or expose them to competitive disadvantages.
Integration of Disparate Data Sources
Many farmers might collect data from multiple sources and formats. Integrating these disparate datasets can be intimidating and technically challenging. Having standardized procedures for data integration is key.
Lack of Technical Knowledge
The gap in technical knowledge about machine learning in the farming community can hinder the effective use of these technologies. Training and resources are crucial for empowering farmers to leverage machine learning effectively.
The Future of Agriculture and Machine Learning
The future landscape of agriculture is poised to change dramatically with the continuous development of machine learning technologies. As datasets become more comprehensive and sophisticated, the potential for achieving sustainability and efficiency in agriculture will grow. Some forward-looking trends include:
Increased Use of AI-Driven Tools
More tools are being developed that incorporate AI capabilities, enabling farmers to harness the full power of agriculture datasets for machine learning. These tools can assist in real-time decision-making and enhance overall farm management.
Enhanced Collaboration through Platforms
Online platforms facilitating collaboration among farmers, researchers, and tech companies will become increasingly popular, allowing for richer datasets and shared learning experiences.
Sustainability Focus
As global awareness of sustainable farming practices increases, machine learning models will be crucial in developing techniques that optimize resource use, minimizing environmental impacts while maximizing production.
Conclusion
In summary, the integration of machine learning in agriculture through the use of comprehensive and high-quality datasets is a turning point for the industry. From enhancing yield predictions to improving pest management strategies, the power of agriculture datasets for machine learning is unparalleled. As technology continues to advance, the synergy between agriculture and machine learning is set to pave the way for a more sustainable, efficient, and prosperous future in farming.
At keymakr.com, we are dedicated to empowering businesses through innovative software development solutions tailored to the agriculture sector. We understand the burgeoning potential of machine learning applications and are here to help you navigate this transformative journey. Embrace the future of agriculture with data-driven insights and cutting-edge technologies.
agriculture dataset for machine learning