The Role of Automatic Image Annotation in Modern Business
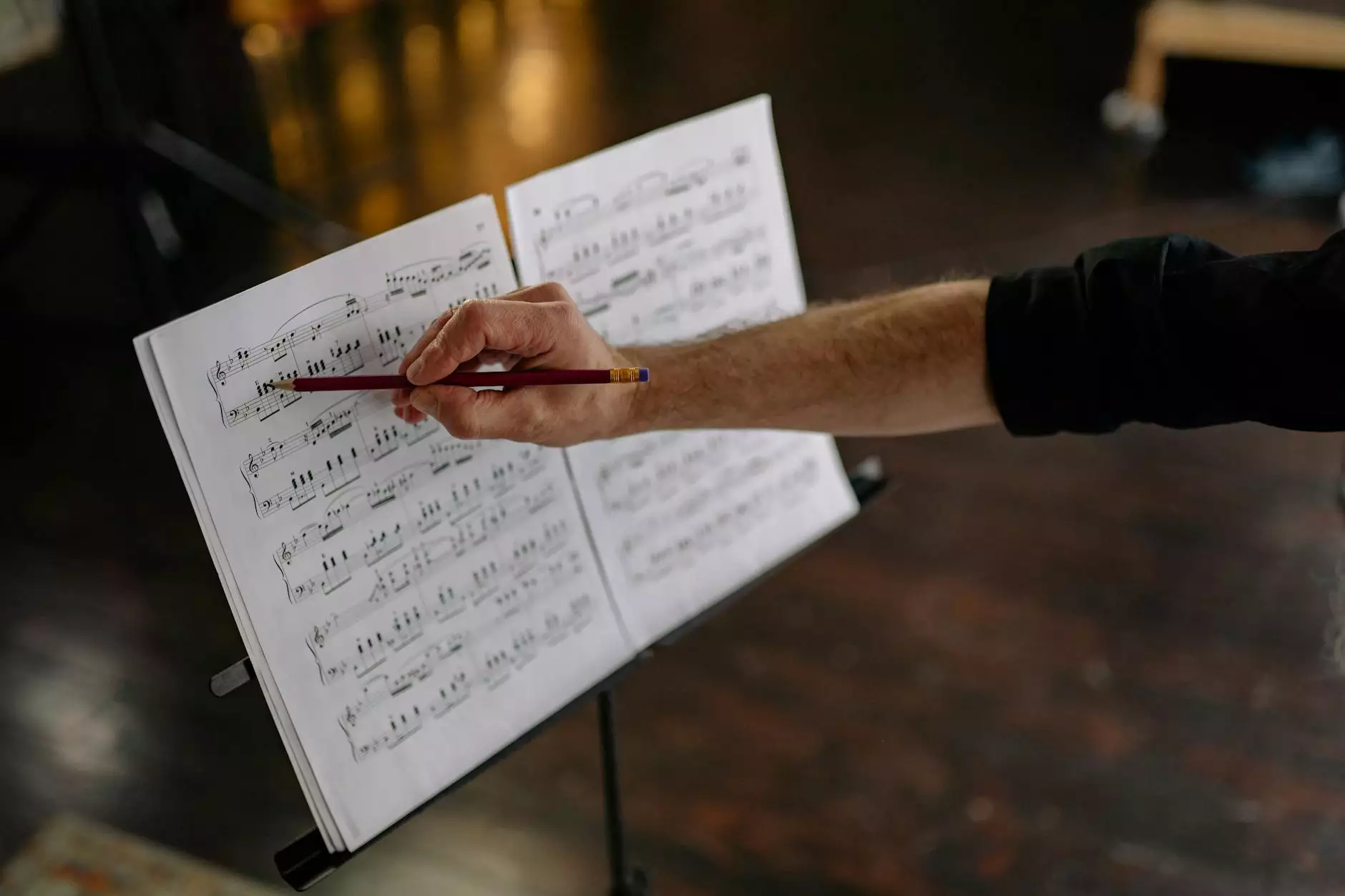
Automatic image annotation is an innovative technology that empowers businesses across various sectors by streamlining their data processing workflows. As the world becomes increasingly data-driven, the importance of effective data annotation cannot be overstated. This article delves into how automatic image annotation functions, its significance for businesses, and how it can enhance operational efficiency.
Understanding Automatic Image Annotation
In essence, automatic image annotation refers to the automatic labeling of images using machine learning algorithms and artificial intelligence (AI). This process involves training models to recognize, classify, and label images based on their visual content.
With advancements in deep learning and computer vision, businesses can now automate the previously labor-intensive task of annotating images. This automation not only saves time but also significantly increases the accuracy and reliability of data labeling.
Why is Automatic Image Annotation Important for Businesses?
In today’s fast-paced business environment, efficient data handling is crucial. Here are several reasons why automatic image annotation is essential:
- Enhanced Efficiency: By automating the annotation process, businesses can process vast volumes of image data in a fraction of the time it would take manually.
- Improved Accuracy: Manual annotation can introduce human errors. Machine learning models, once trained, provide consistent and highly accurate annotations.
- Cost Reduction: Reducing the time and effort spent on data annotation leads to significant cost savings, enabling businesses to allocate resources more effectively.
- Scalability: As businesses grow, so does their data. Automatic annotation tools can scale with business needs, accommodating larger datasets without a hitch.
- Data-Driven Decision Making: With accurate annotations, companies can derive meaningful insights from their data, facilitating informed decision-making.
How Automatic Image Annotation Works
The process of automatic image annotation involves several key components:
1. Image Acquisition
The first step is acquiring images that need to be annotated. This can include images from various sources, such as cameras, scanners, or images already stored in databases.
2. Pre-processing
Before annotation can take place, images often undergo pre-processing. This step cleans and organizes the data, making it easier for machine learning models to perform effectively. Common techniques include resizing, normalization, and format conversion.
3. Training Machine Learning Models
The core of automatic image annotation is the machine learning model. Businesses typically use large, labeled datasets to train these models. Techniques like Convolutional Neural Networks (CNNs) are widely employed for their ability to identify and extract features from images.
4. Annotation Process
Once trained, the model can automatically label new images. The algorithm will analyze visual content, identify key features, and apply appropriate labels based on learned patterns.
5. Post-processing
After annotation, a post-processing step can help refine the results. This may involve filtering out incorrect annotations or applying additional verification steps to enhance overall accuracy.
Applications of Automatic Image Annotation in Business
There is a wide array of applications for automatic image annotation across industries. Below is a closer look at how different sectors benefit:
1. Retail and E-commerce
In retail, automatic image annotation helps categorize products for online stores. By analyzing product images, businesses can automatically assign tags and optimize search functionality within their e-commerce platforms. This leads to a better customer experience and increased sales conversions.
2. Automotive Industry
The automotive sector utilizes automatic image annotation to improve autonomous vehicle systems. By effectively labeling images from cameras on vehicles, manufacturers can train AI systems to recognize road signs, pedestrians, and other vehicles, enhancing safety and navigation capabilities.
3. Healthcare
In healthcare, automatic image annotation plays a crucial role in analyzing medical images. By annotating X-rays, MRIs, or CT scans, AI models can assist doctors in diagnosing conditions earlier and more accurately, thereby improving patient outcomes.
4. Agriculture
Agricultural businesses leverage automatic image annotation to monitor crop health through drones and satellite imagery. By categorizing images based on plant health, farmers can make data-driven decisions about resource allocation, pest control, and harvest planning.
5. Security and Surveillance
For security applications, automatic image annotation helps in identifying suspicious activities or tracking individuals in surveillance footage. This technology improves safety measures across public and private spaces.
Choosing the Right Automatic Image Annotation Tool
With numerous tools available in the market, it’s crucial for businesses to choose the right Data Annotation Tool or Data Annotation Platform. Consider the following factors:
- Accuracy: The effectiveness of the tool in delivering accurate annotations is paramount. Look for solutions that leverage advanced AI algorithms.
- User-Friendliness: A user-friendly interface enables teams to leverage the tool efficiently, even if they lack deep technical expertise.
- Integration Capabilities: Ensure the annotation tool integrates seamlessly with your existing data pipelines and workflows.
- Scalability: The tool should be able to grow alongside your business needs, accommodating larger datasets as you expand.
- Support and Training: A reputable provider should offer robust support and training resources to help users maximize the tool's potential.
Conclusion
The emergence of automatic image annotation signifies a monumental shift in how businesses handle image data. By leveraging this technology, organizations can not only enhance their operational efficiency but also harness the power of data to drive strategic decision-making.
As the demand for precise and efficient data annotation continues to grow, investing in automation tools becomes not just beneficial but essential. Companies like Keylabs.ai offer cutting-edge solutions that seamlessly integrate automatic image annotation, positioning businesses for success in an increasingly competitive landscape.
Ultimately, embracing automatic image annotation is a forward-thinking step towards innovation, efficiency, and excellence in data processing. With the right tools and strategies, businesses can transform not only their internal processes but also their market positioning.