Unlocking Business Potential with Data Labeling in Machine Learning
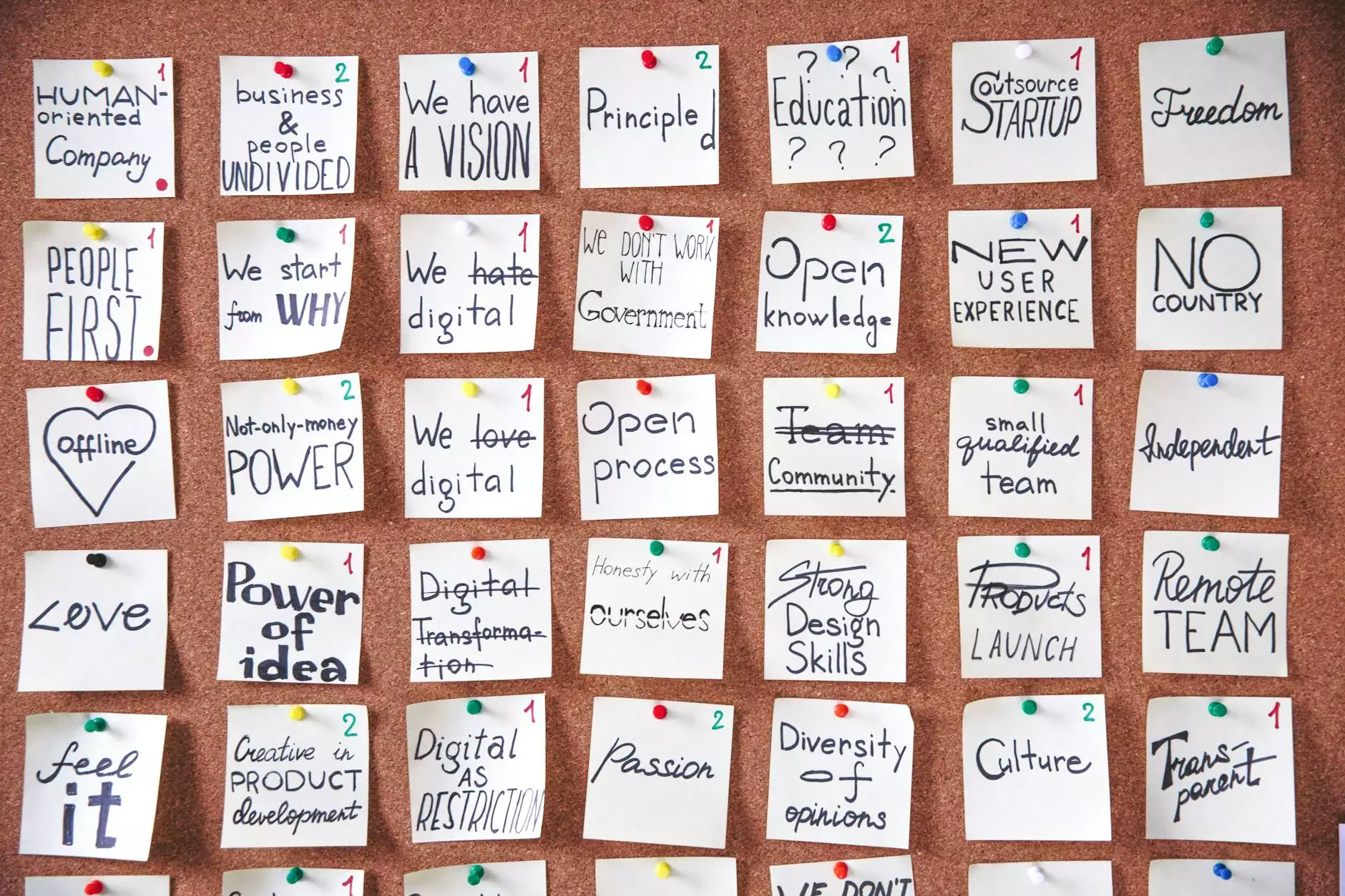
Understanding Data Labeling in Machine Learning
Data labeling is a crucial aspect of the machine learning pipeline that involves tagging or annotating data so that a machine learning model can learn from it. The process typically requires human input to categorize data accurately, which can range from images, text, audio, and even video. In sectors like Home Services and Keys & Locksmiths, data labeling can significantly enhance business operations, customer services, and marketing strategies.
Why is Data Labeling Important?
The significance of data labeling in machine learning cannot be overstated. Here are several reasons why data labeling is fundamental for businesses:
- Improved Accuracy: Well-labeled data ensures that machine learning models can make precise predictions, reducing errors in applications such as customer support and service optimization.
- Enhanced Training: Data labeling is essential for training supervised learning algorithms, which rely on labeled datasets to learn and improve their accuracy over time.
- Better Insights: Labeled data allows businesses to extract valuable insights, helping them understand customer behaviors and trends within the Home Services and Locksmith markets.
- Efficient AI Deployment: Accurate labeling speeds up the deployment of AI solutions, allowing companies to implement machine learning tools quickly and effectively.
How Data Labeling Works
Data labeling involves several critical steps, each designed to ensure that the data is appropriately tagged for machine learning applications:
- Data Collection: Gather raw data from various sources, ensuring diversity and volume to create a representative dataset.
- Labeling Guidelines: Establish clear labeling criteria that annotators will follow to maintain consistency across all data samples.
- Annotation: Human annotators (or automated tools) tag the data according to the established guidelines, converting raw data into labeled datasets.
- Quality Control: Implement a quality assurance process to verify the accuracy of the labeled data, which may involve a second round of reviews.
- Feedback and Iteration: Use feedback from the model’s performance to refine the data labeling process and improve quality over time.
The Role of Data Labeling in Home Services and Keys & Locksmiths
In the context of Home Services and Keys & Locksmiths, effective data labeling can transform how businesses interact with their customers. Here are a few applications:
1. Customer Interaction and Support
By utilizing machine learning models trained on well-labeled customer interaction data, businesses can enhance their customer support systems. For instance, natural language processing (NLP) models can analyze customer inquiries and efficiently route them to the appropriate service team, ensuring rapid responses and higher satisfaction rates.
2. Predictive Maintenance
Labeled data from service calls helps create predictive maintenance models. For example, tagging service incidents based on machine performance allows businesses to predict when a customer’s locks or security systems may require maintenance, leading to proactive service offerings.
3. Marketing Strategies
Data labeling enhances the ability to segment customers based on their behaviors and preferences. Utilizing labeled data, machine learning algorithms can identify customer patterns that guide targeted marketing campaigns, ensuring they reach the right audience with the right message at the right time.
Benefits of Utilizing Data Labeling Services
Investing in a professional data labeling service can offer your business numerous advantages:
- Expertise: Professional data labelers bring experience and a keen understanding of best practices in tagging data, ensuring higher quality outputs.
- Scalability: Outsourcing labeling can help businesses scale operations without the need for hiring and training additional in-house staff.
- Cost-Effective: It often proves more economical to work with external providers than managing an internal team, especially when handling large datasets.
- Time Savings: With dedicated resources focused on data labeling, businesses can accelerate their machine learning projects and get to market faster.
Choosing the Right Data Labeling Partner
When considering a data labeling service provider, here are several factors to evaluate:
- Experience and Track Record: Look for a provider with a proven history in your industry that can demonstrate successful partnerships with similar companies.
- Quality Control Processes: Ensure the provider has robust quality assurance measures to maintain high accuracy rates in labeled data.
- Technology and Tools: Check if they utilize the latest technologies and tools for data labeling, which can enhance efficiency and effectiveness.
- Cost Structure: Compare the pricing models of different service providers to find a solution that meets your business budget while offering quality services.
- Scalability: Ensure the partner can grow with your business, adapting to your needs as you gather more data.
Common Challenges in Data Labeling
While data labeling is essential, it’s not without its challenges:
- Subjectivity: Different annotators may interpret labeling tasks differently, leading to inconsistencies.
- Volume of Data: Large datasets can overwhelm data labeling teams, causing delays and potential errors in the process.
- Resource Intensive: Data labeling can be time-consuming and labor-intensive, requiring careful allocation of resources to ensure timely completion.
- Quality Assurance: Maintaining high-quality labeling over time can be challenging, particularly for complex datasets.
Future Trends in Data Labeling in Machine Learning
As machine learning continues to evolve, several trends may influence the field of data labeling:
- Automation: The advancement of AI tools for automated data labeling is expected to grow, although these tools will still require human oversight.
- Remote Teams: The increasing acceptance of remote work will lead to more distributed teams specializing in data labeling, enhancing flexibility and access to talent.
- Ethical Considerations: As machine learning applications expand, businesses will need to focus on ethical data practices, including fairness and bias in labeled datasets.
- Real-time Labeling: Innovations in technology may enable real-time data labeling, critical for industries requiring instantaneous data processing.
Conclusion: Leverage Data Labeling for Business Success
For businesses operating in the Home Services, Keys & Locksmiths sectors, understanding and implementing effective data labeling strategies is key to harnessing the power of machine learning. By investing in quality data labeling, you position your business for greater accuracy, enhanced customer experiences, and ultimately, increased profitability. In a world driven by data, the effectiveness of data labeling in machine learning can unlock new opportunities that help you stay ahead of the competition.
Call to Action
Are you ready to transform your business with data labeling in machine learning? Contact KeyMakr.com today to discover how our expert services can enhance your operations and drive growth.
data labeling machine learning